Projects
Responsible, Explainable, and Fair AI (REF-AI) for Medical Imaging
Integrating responsible, explainable, and fair artificial intelligence (REF-AI) into medical image analysis has gained significant attention in recent years. This has been driven by the pressing need for ethical, trustworthy, and transparent implementation of AI systems in healthcare. The following review provides a concise overview of REF-AI in the context of medical image analysis.
Read more ...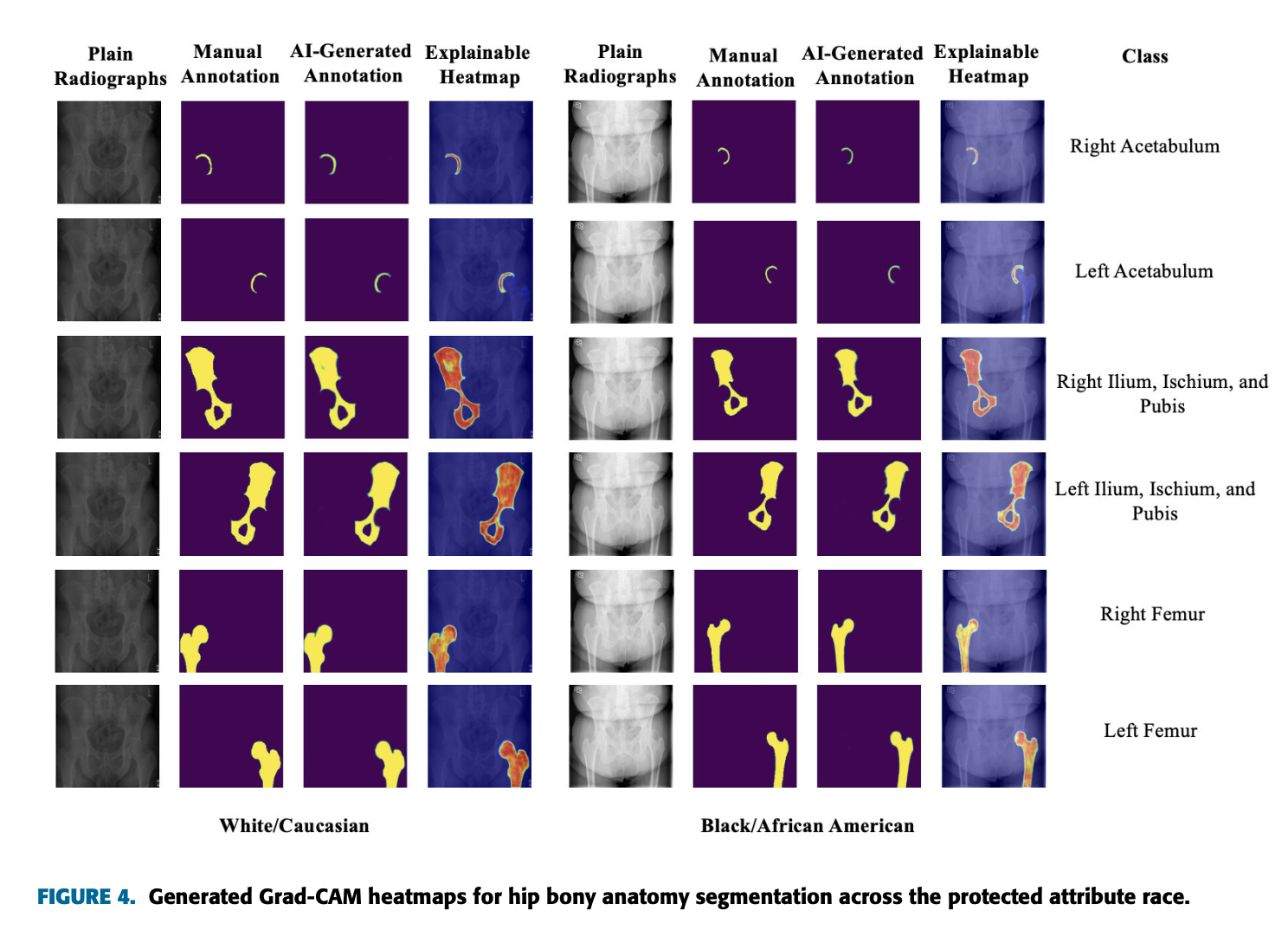
Performance of Cementless Hip Arthroplasty Stem Types Based on Consolidated Large Registry Data
Improvements in cementless total hip arthroplasty have been directed at optimizing osseointegration of the femoral implant to reduce aseptic loosening rates. Stem design plays a critical role in the performance of these implants. Given the increase in new stem designs and the creation of an updated classification system, improved understanding of the outcomes of each stem type is warranted. The purpose of this study was to determine overall revision rates based on stem design and proprietary model.
Read more ...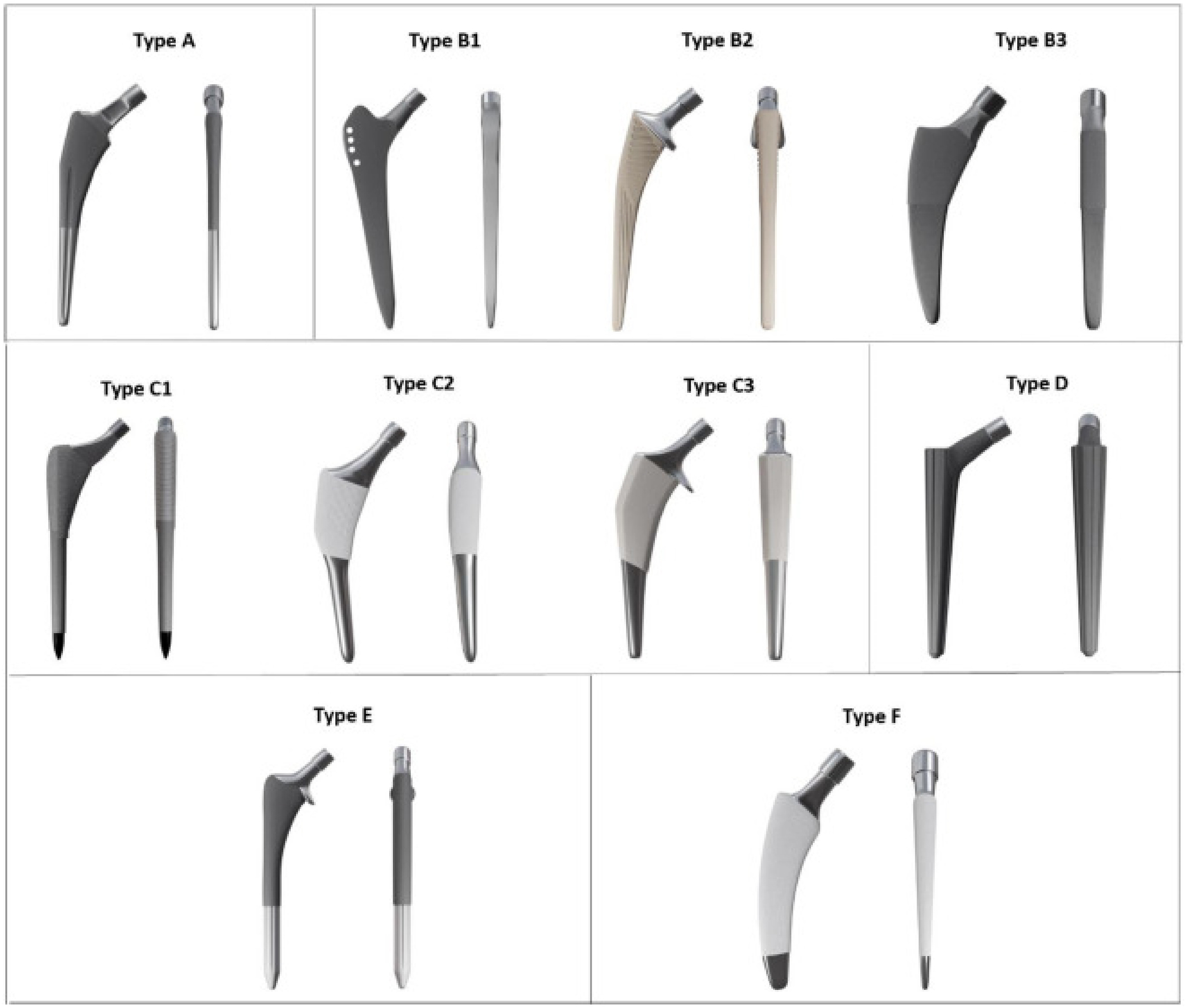
Explainable Feature Engineering in Health Data Science
Machine learning (ML) is demonstrating remarkable success in various healthcare applications. The success of ML in healthcare is inherently linked to the rigorous process of feature engineering and feature selection, which truly forms the backbone of ML model development. This study investigates the role of a well-known large language model (LLM), the ChatGPT-4o, in feature selection and classification processes for healthcare data, focusing on the explainability of ML.
Read more ...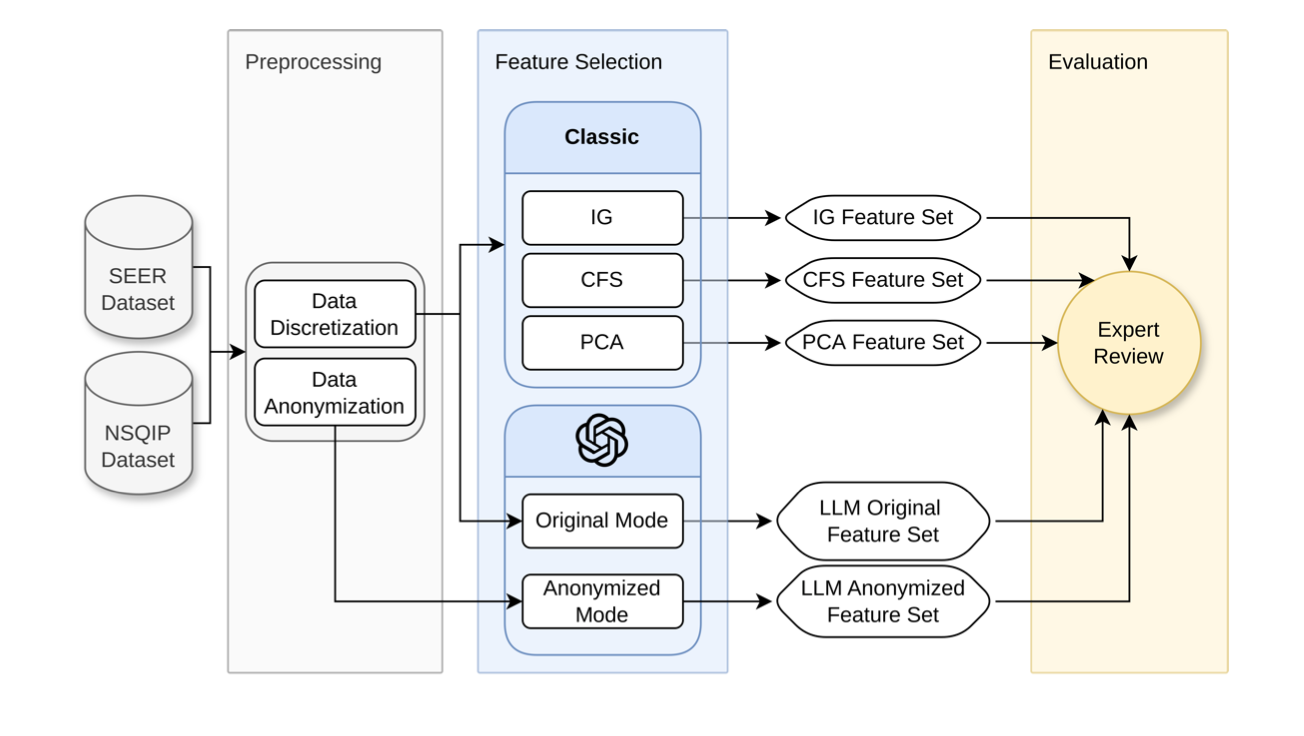
Fair and Explainable AI for Cancer Survival Prediction
While prior machine learning (ML) models for cancer survivability prediction often treated all cancer stages uniformly, cancer survivability prediction should involve understanding how different stages impact the outcomes. Additionally, the success of ML-powered cancer survival prediction models depends a lot on being fair and easy to understand, especially for different stages of cancer. This study addresses cancer survivability prediction using fair and explainable ML methods.
Read more ...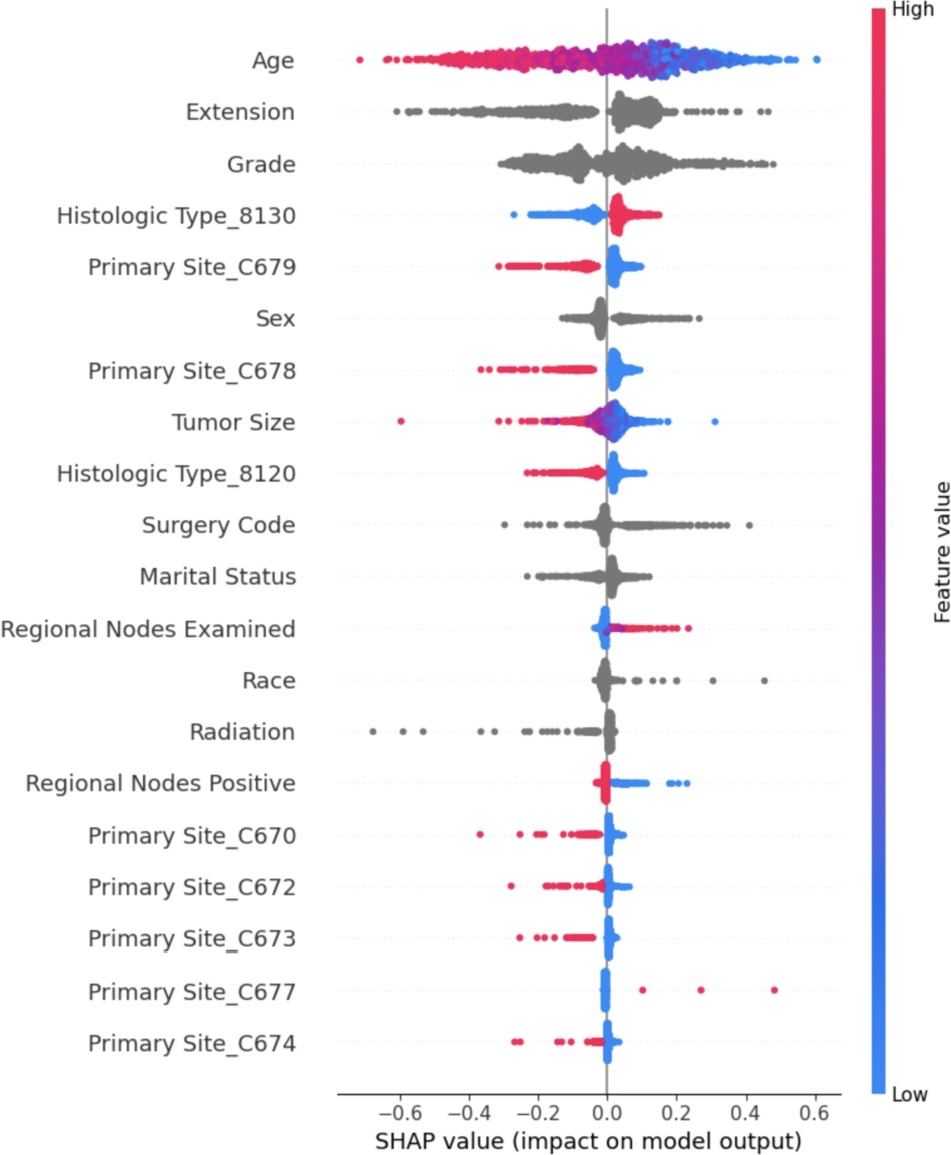
Generative AI in Orthopedics Imaging
Deep learning medical image analysis in orthopedics has gained momentum in recent years, but progress has been limited by the absence of large-scale, standardized ground-truth datasets. This study introduces a deep few-shot image augmentation pipeline that synthetically generates knee radiographs to support downstream tasks such as KL grading for knee osteoarthritis.
Read more ...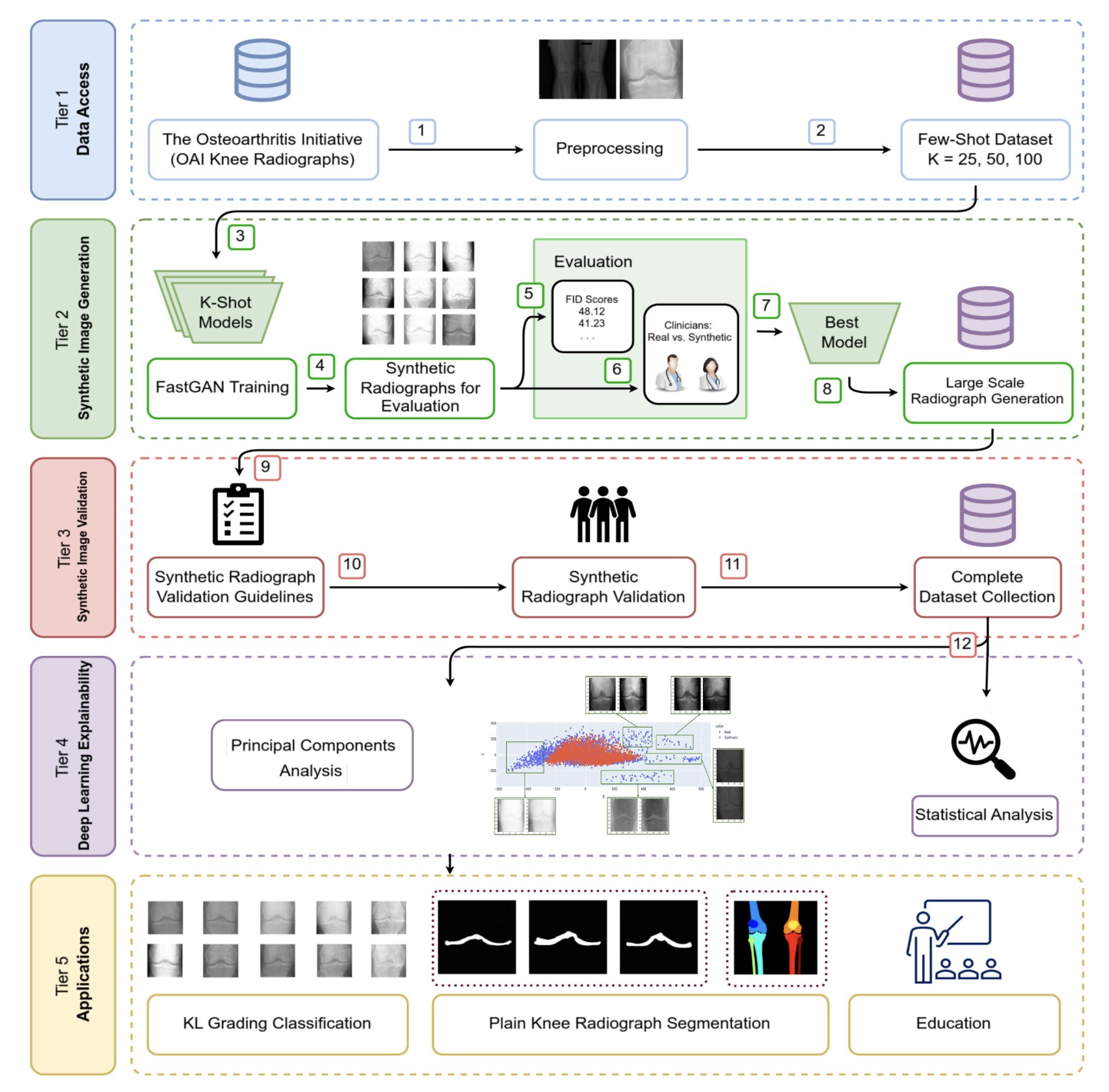
Advancing AI-Powered Orthopedic Image Segmentation: Mitigating Bias for Enhanced and Equitable Healthcare.
AI-powered segmentation of hip and knee bony anatomy has revolutionized orthopedics, transforming both pre-operative planning and post-operative assessment. However, despite the remarkable advancements in deep learning computer vision algorithms for medical image segmentation, a critical issue remains largely unexplored: the potential for biases inherent within these AI-powered models.
Read more ...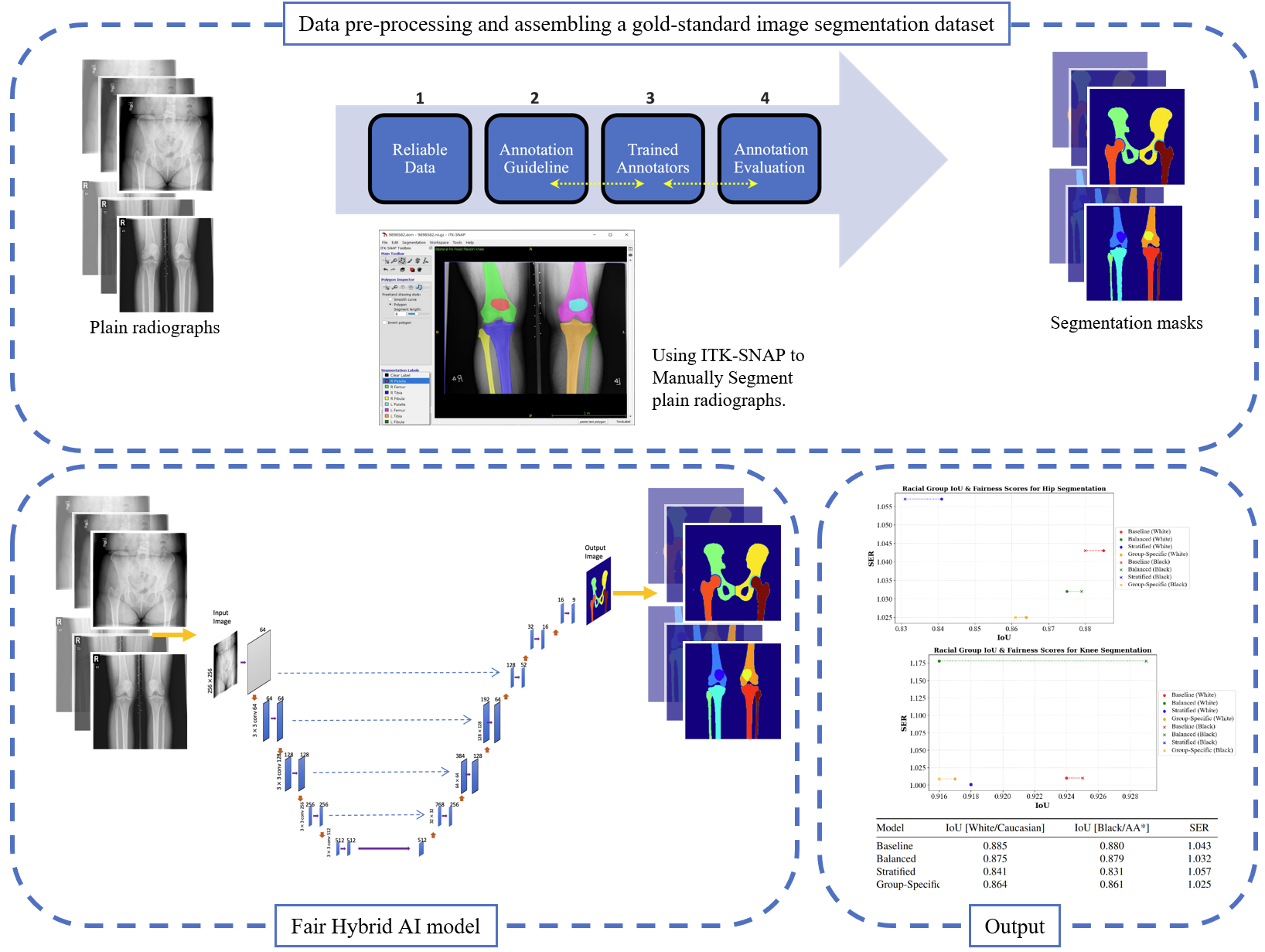
Acquiring Impartial Image Segmentation Skills: An In-depth Analysis Using Plain Knee Radiographs as a Case Study
Automatic segmentation of knee bony anatomy is essential in orthopedics, and it has been around for several years in both pre-operative and post-operative settings. While deep learning algorithms have demonstrated exceptional performance in medical image analysis, the assessment of fairness and potential biases within these models remains limited. This study aims to revisit deep learning-powered knee-bony anatomy segmentation using plain radiographs to uncover visible gender and racial biases.
Read more ...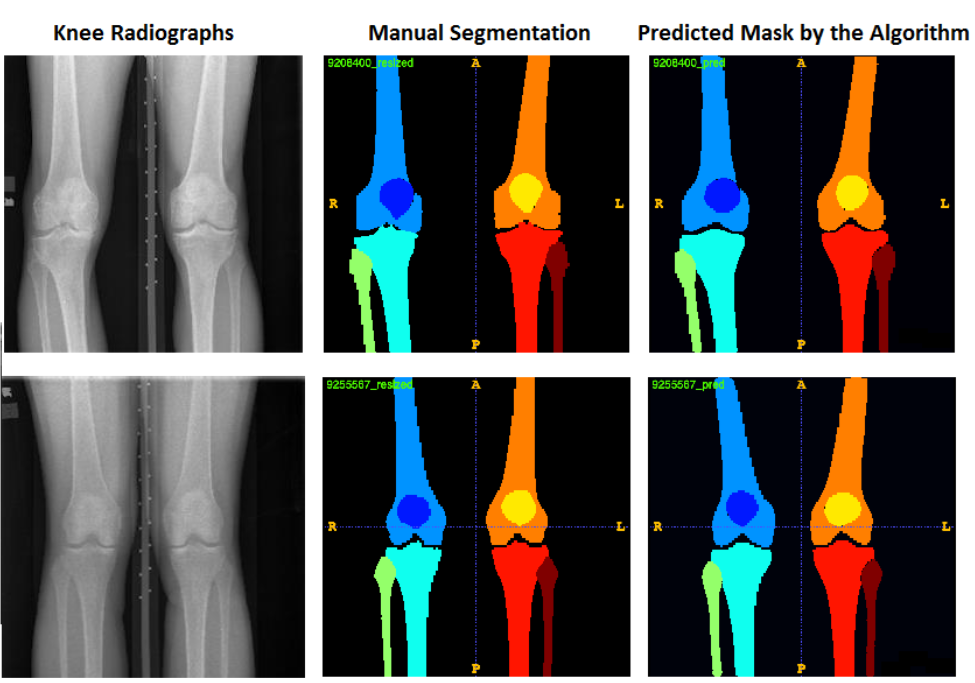
A Textual Dataset Designed to Promote Progress in Open Scientific Research in Total Joint Arthroplasty (TJA)
Total joint arthroplasty (TJA) is the most common and fastest inpatient surgical procedure in the elderly, nationwide. Due to the increasing number of TJA patients and advancements in healthcare, there is a growing number of scientific articles being published in a daily basis. These articles offer important insights into TJA, covering aspects like diagnosis, prevention, treatment strategies, and epidemiological factors.
Read more ...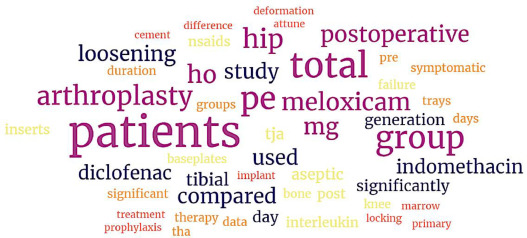
An Ensemble Machine Learning Method to Predict Unplanned Return to Operating Room Following Primary Total Shoulder Arthroplasty
Reoperation is the most significant compilation following any surgical procedure. Developing machine learning methods that can predict the need for reoperation will allow for improved shared surgical decision making, and patient-specific and preoperative optimization. Yet, no precise machine learning models have been published to perform well in predicting the need for a reoperation within 30-days following primary total shoulder arthroplasty (TSA). This study aimed to build, train, and evaluate an ensemble machine learning method that predicts return to the operating room following primary TSA.
Read more ...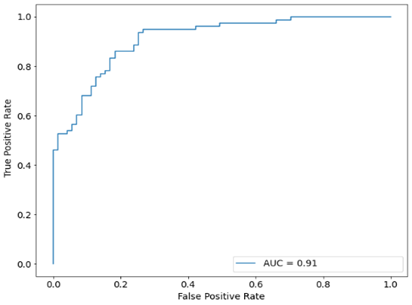
Smart Mobile Phone Applications for Measuring Knee Range of Motion
Knee range of motion is an important indicator of function in both the native and surgically replaced knee. In the clinical setting, range of motion can be adequately evaluated by the surgeon either visually or with the assistance of a goniometer. Comparatively, patients may not be as experienced in estimating their knee range of motion (ROM) outside of clinic or the hospital. Developing a mobile application through which patients can easily assess their knee ROM may provide a more accessible tool for measuring progress in regaining function. The purpose of this study was to validate a mobile phone application developed to measure knee ROM, when compared to visual-based and goniometer-based ROM measurements obtained in an Orthopedic clinic setting.
Read more ...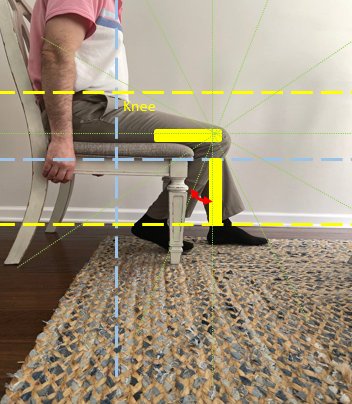
Enforcing Explainable Deep Few-Shot Learning to Analyze Plain Knee Radiographs
This work is the first to present an explainable deep few-shot learning model that can localize the knee joint area and segment the joint space in plain knee radiographs, using only a small number of manually annotated radiographs. The accuracy performance of the proposed method was thoroughly and experimentally evaluated using various image localization and segmentation measures, and it was compared to baseline models that utilized large-scale fully-annotated training datasets.
Read more ...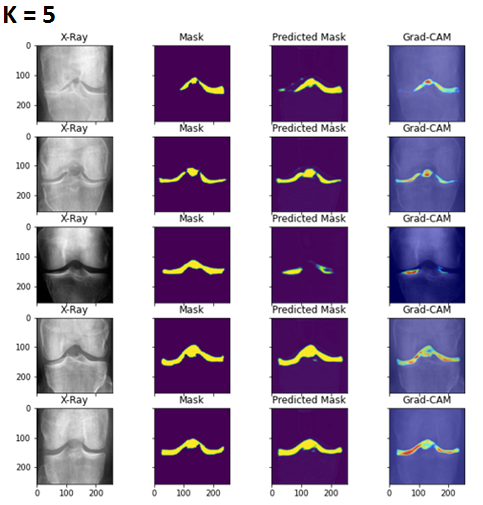
Word Embedding Neural Networks to Advance Knee Osteoarthritis Research
Knee OA is not a curable disease yet, and it affects large columns of patients, making it costly to patients and healthcare systems. Although knee OA carries a list of well-known terminology aiming to standardize the nomenclature of the diagnosis, prognosis, treatment, and clinical outcomes of the chronic joint disease, in practice there is a wide range of terminology associated with knee OA across different data sources, including but not limited to biomedical literature, clinical notes, healthcare literacy, and health-related social media. Among these data sources, the scientific articles published in the biomedical literature usually make a principled pipeline to study disease. The present works aim to utilize artificial neural network strategies to automatically extract vocabularies associated with knee OA diseases using scientific articles.
Read more ...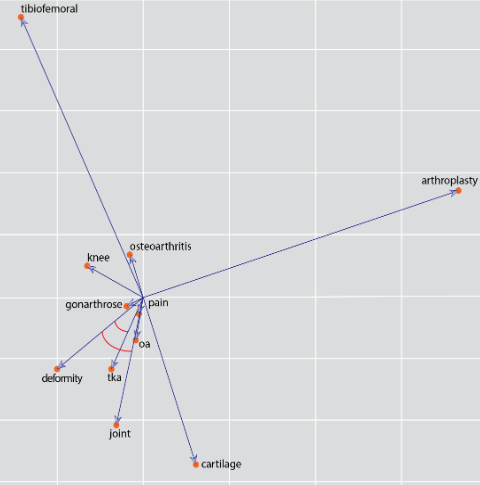
Give Me a Knee Radiograph, I Will Show You Where the Knee Joint Space Is: Enforcing Few-Sample Learning for Knee Semantic Segmentation
From the computational perspective, deep learning computer vision methods have already demonstrated very successful applications in a variety of medical image analysis tasks. However, there are several fundamental challenges that stop deep learning methods to obtain their full potential in healthcare settings. One can see that they often need a large column of annotated training data to achieve better accuracy over traditional machine learning methods. The current work tends to use deep few-shot learning to tackle the problem of knee joint space segmentation in plain radiographs using only few samples of manually segmented radiographs.
Read more ...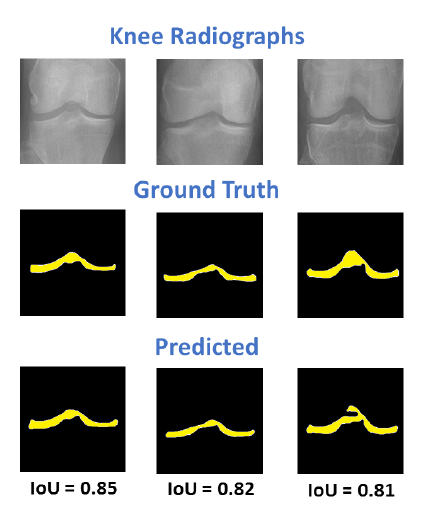
Give Me a Knee Radiograph, I Will Tell You Where the Knee Joint Area Is
Knee pain is clinically evaluated by routine radiographs, where the widespread adoption of radiographic images and their availability at low cost, make them the principle component in the assessment of knee pain and knee pathologies. The current work proposes an accurate and effective pipeline for autonomous detection, localization, and classification of knee joint area in plain radiographs combining the You Only Look Once (YOLO v3) deep convolutional neural network with a large and fully-annotated knee radiographs dataset.
Read more ...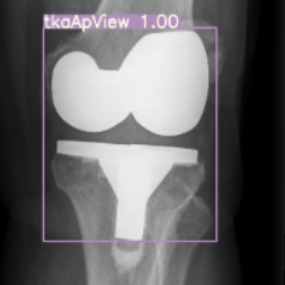
DeepTKAClassifier: Brand Classification of Total Knee Arthroplasty Implants using Explainable Deep Convolutional Neural Networks
Total knee arthroplasty (TKA) is one of the most successful surgical procedures worldwide. It improves quality of life, mobility, and functionality for the vast majority of patients. However, a TKA surgery may fail over time for several reasons, thus it requires a revision arthroplasty surgery. Identifying TKA implants is a critical consideration in preoperative planning of revision surgery. This study aims to develop, train, and validate deep convolutional neural network models to precisely classify four widely-used TKA implants based on only plain knee radiographs. Using 9,052 computationally annotated knee radiographs, we achieved weighted average precision, recall, and F1-score of 0.97, 0.97, and 0.97, respectively, with Cohen Kappa of 0.96.
Read more ...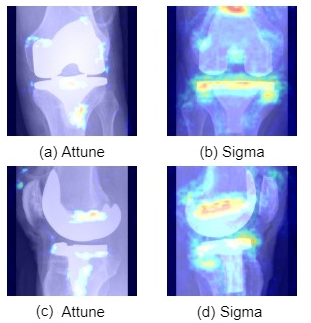
NLP-Powered Algorithms to Identify Common Data Elements in Operative Notes for Knee Arthroplasty
Within a cohort of 20,000 knee arthroplasty operative notes from 2000 to 2017 at a large tertiary institution, we randomly selected independent pairs of training and test sets to develop and evaluate NLP algorithms to detect five major data elements. The size of the training and test datasets were similar and ranged between 420 to 1592 surgeries. Expert rules using keywords in operative notes were used to implement NLP algorithms capturing: (1) category of surgery (total knee arthroplasty, unicompartmental knee arthroplasty, patellofemoral arthroplasty), (2) laterality of surgery, (3) constraint type, (4) presence of patellar resurfacing, and (5) implant model (catalog numbers). We used institutional registry data as our gold standard to evaluate the NLP algorithms. It achieved 98.3%, 99.5%, 99.2%, and 99.4% accuracy on test datasets, respectively. The implant model algorithm achieved an F1-score of 99.9%.
Read more ...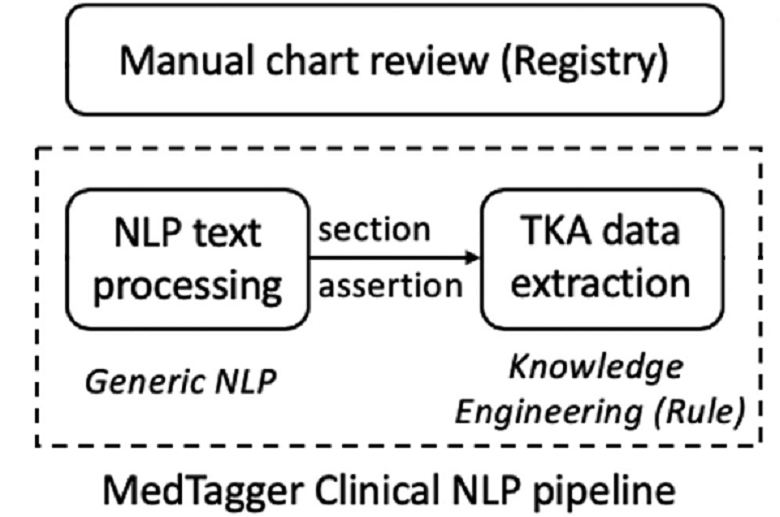