Predicting Cancer Survival by Stage Using Explainable ML
While prior machine learning (ML) models for cancer survivability prediction often treated all cancer stages uniformly, effective survivability prediction should account for how different stages impact outcomes. Moreover, the success of ML-powered models relies heavily on fairness and interpretability, especially across varying cancer stages. This study addresses cancer survivability prediction using fair and explainable ML approaches. Focusing on bladder, breast, and prostate cancers using SEER Program data, we developed and validated stage-specific ML models designed to enhance fairness and transparency. Our computational strategies emphasize both model performance and the ethical imperative of equitable and interpretable care. The findings underscore the importance of ML fairness and explainability in capturing and interpreting key factors influencing cancer survivability. Overall, this work advocates for integrating fairness and explainability into ML models to enable equitable, transparent, and clinically useful predictions, ultimately improving patient care and supporting shared decision-making in oncology.
GitHub: ML_Fairness_Explainability_Cancer_Survivability
Paper: Published in ScienceDirect
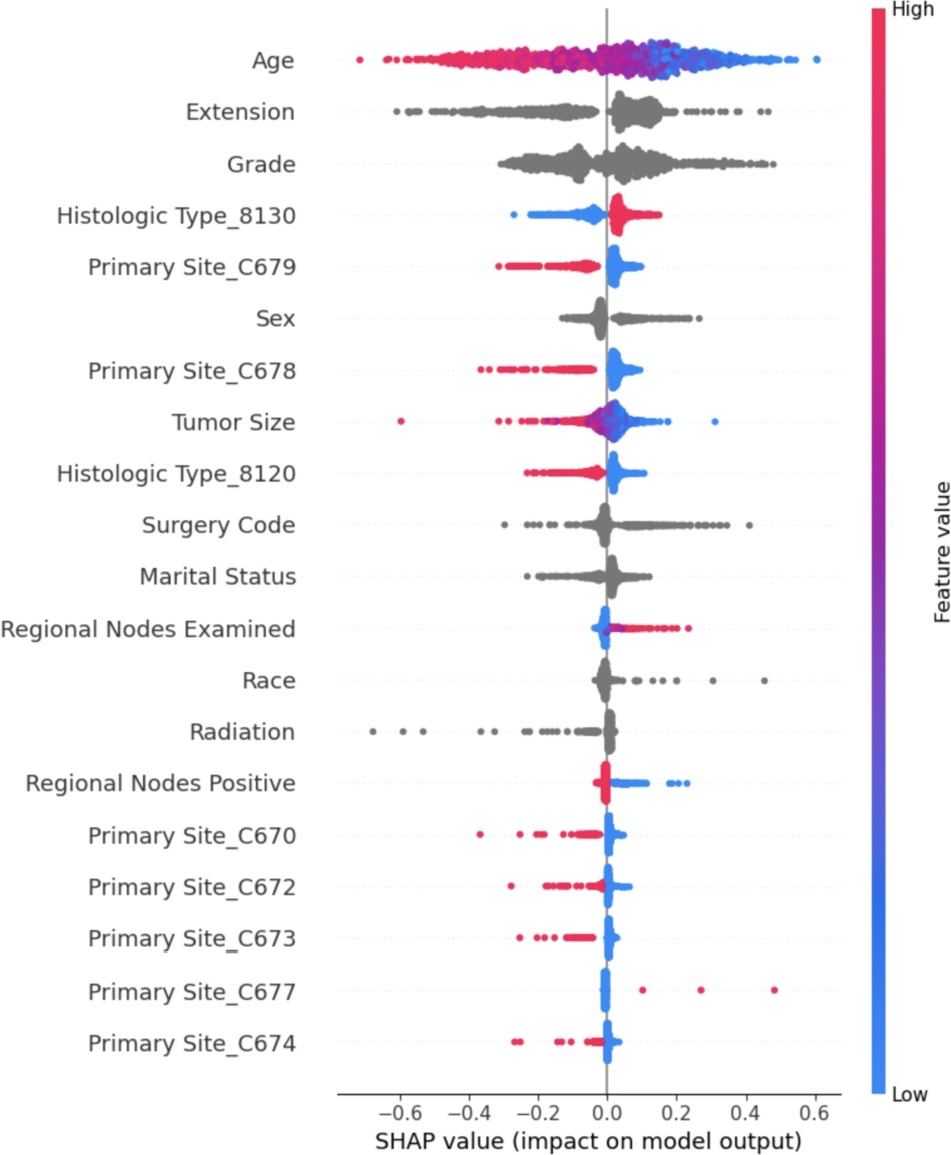